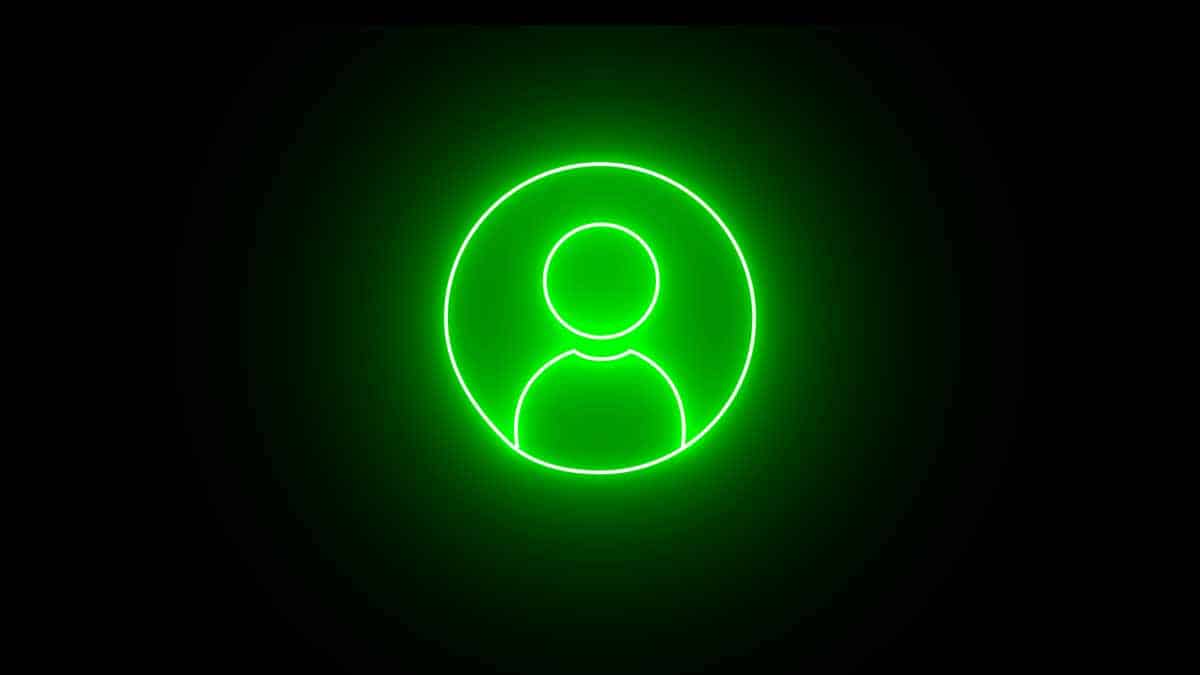
Jump to a section:
In the realm of modern marketing, few strategies are as impactful as predictive user segmentation. By grouping users based on their behaviors, preferences, and predicted future actions, businesses can deliver hyper-targeted content, offers, and services that significantly boost engagement and ROI. Now, with the rise of generative AI, this approach has become not just more efficient, but more insightful. In this guide, we’ll explore how generative AI can amplify your predictive user segmentation efforts, the steps required to implement it, and the challenges you might face along the way.
1. Understanding Predictive User Segmentation
What Is Predictive User Segmentation?
In simplest terms, predictive user segmentation leverages data science and machine learning models to categorize users into segments based on their predicted future behaviors. These behaviors could include the likelihood of purchase, churn risk, engagement patterns, and more. Traditional segmentation might rely on static attributes (e.g., age, location), while predictive segmentation goes a step further by using algorithms to anticipate what a user might do next.
Why Generative AI?
Generative AI adds a new twist to this process by helping you generate more nuanced insights. Traditional machine learning approaches generally require clearly defined labels, structured datasets, and explicit feature engineering. Generative AI models, such as GPT-based systems, can “understand” unstructured data—like text from social media posts, customer feedback, or support tickets—and generate meaningful insights or predictions. They can also suggest segment definitions, write segment descriptions, and even propose marketing strategies tailored to each segment.
A few notable stats to illustrate the growing importance of AI in segmentation:
- A 2024 Gartner report predicts that over 75% of B2C marketers will adopt some form of AI-based segmentation in their marketing stack.
- According to Deloitte, organizations that use predictive segmentation see an average 20-30% increase in click-through rates on targeted campaigns.
- A survey by McKinsey shows that 38% of marketers cite customer segmentation and personalization as their top priority for AI investments over the next two years.
2. Key Benefits of Generative AI in Predictive Segmentation
Deeper Customer Understanding
Generative AI doesn’t just categorize users based on pre-existing data fields—it can interpret and contextualize complex behavioral and textual data. For instance, an e-commerce platform might have thousands of user reviews. A generative AI model can process these reviews to identify sentiment, recurring complaints, or product attributes that matter most. This helps you form segments based on more than simple demographics.
Dynamic Segment Creation
Segments often need to evolve in real-time as user behaviors shift. With generative AI, you can automate the constant monitoring and updating of user segments. Instead of relying on manual triggers or monthly data refreshes, AI systems can watch user data flows and adjust segment definitions as soon as they detect a pattern shift.
Predictive Precision
By incorporating multiple data sources—such as past purchases, website visits, email interactions, and even unstructured text—generative AI can offer highly accurate predictions about which users will be most receptive to certain campaigns. This can reduce wasted ad spend and improve conversion rates.
Creative Insights and Campaign Ideas
Because generative AI models can also generate text content, they can go beyond the numbers. They might propose campaign taglines, email subject lines, or even potential offers tailored to each segment. This capacity frees up your marketing team from mundane tasks, allowing them to focus on strategy and innovation.
3. The Process: How to Implement Generative AI for Predictive User Segmentation
Step 1: Define Your Segmentation Goals
- Clarify Your Objectives
Are you trying to increase sales, reduce churn, or boost user engagement with a specific feature? Knowing your target outcome helps you steer the AI in the right direction. - Identify Relevant KPIs
Common metrics might include customer lifetime value (CLV), churn rate, average order value (AOV), or conversion rate. Decide which metrics define “success” for your segmentation efforts.
Step 2: Gather and Prepare Your Data
- Collect Data from Multiple Sources
Pull in CRM data, web analytics, purchase histories, and user feedback. If you want to leverage generative AI’s text-processing capabilities, be sure to include unstructured data like reviews, chat transcripts, or survey responses. - Cleanse and Format
Remove duplicate records, fix inconsistent data formats, and address missing or incomplete fields. Generative AI models thrive on clarity, so garbage in, garbage out is as relevant as ever. - Segment Data by Relevancy
Not all data is helpful. If you’re focused on churn prediction, for instance, look for data points that historically correlate with churn (e.g., last login date, subscription renewal dates).
Step 3: Choose a Generative AI Model
- Pre-Trained vs. Fine-Tuned
You might start with a pre-trained model like GPT or Bloom. For improved accuracy, particularly for industry-specific jargon or specialized user behaviors, consider a fine-tuned model trained on your own data. - APIs and Integrations
Many generative AI providers offer APIs that let you seamlessly integrate the model with your existing tools (e.g., CRMs like HubSpot, Salesforce). Pick solutions that align with your tech stack.
Step 4: Develop Predictive Segmentation Logic
- Feature Engineering
Identify the variables that your AI model should weigh. For instance, if you’re forecasting who might upgrade to a premium membership, key features might include time spent on the platform, number of premium features used, and frequency of interactions with customer support. - Model Training
Use historical data to train the model. You can feed it examples of customers who took certain actions (e.g., purchased, unsubscribed) and have the AI discern the patterns that differentiate them from those who did not. - Validation
Split your data into a training set and validation/test set to see how well the model generalizes. Evaluate metrics like precision, recall, and F1 score if you’re predicting user actions. This step ensures the model isn’t just memorizing historical patterns but is truly learning to predict.
Step 5: Generate Segments and Insights
- Automated Grouping
Allow the AI to propose various segment definitions. For instance, it might cluster users by predicted purchase frequency, product interest, or sentiment expressed in reviews. - Generative Analysis
Ask the AI to summarize the rationale behind each segment. For example, “Users in Segment A tend to visit the website on weekends and often purchase higher-value items after reading 3-5 product reviews.” - Human Review
Review the generated segments to ensure they make sense. Sometimes, AI might form segments that are too broad or too obscure. Collaborate with your marketing or product teams to refine them.
Step 6: Deploy and Monitor
- Integration with Marketing Automation
Once you have well-defined segments, feed these into your marketing automation system to deliver personalized emails, ads, or website experiences. - Real-Time Updates
Ideally, your generative AI model is set up for continuous or periodic re-training. This means segments get updated automatically as new data comes in. - Performance Tracking
Monitor how well each segment performs with your campaigns. For instance, you might see that Segment A yields a 15% increase in email open rates, while Segment B leads to a 20% lift in conversions. Use these insights to further refine your segmentation strategy.
4. Challenges and How to Overcome Them
Data Privacy and Compliance
- Challenge: Handling personal data can be risky, especially if you’re operating in regions with strict data protection laws (e.g., GDPR in Europe).
- Solution: Anonymize or pseudonymize user data. Use on-premise or private cloud models if needed. Confirm that your AI vendor complies with relevant regulations.
Bias in Models
- Challenge: Generative AI models can inadvertently learn biases from training data, resulting in skewed or discriminatory segment definitions.
- Solution: Regularly audit the segments for unusual biases (e.g., focusing disproportionately on certain demographics). Use diverse training data and incorporate bias detection tools.
Overfitting and Inaccuracies
- Challenge: If the model has “memorized” specific patterns that aren’t generally true, your predictive accuracy will drop when applied to new data.
- Solution: Maintain a proper training-validation split, perform cross-validation, and keep track of performance metrics in real-time. Employ early stopping or regularization techniques to combat overfitting.
Resource Constraints
- Challenge: Training and deploying advanced AI models can be resource-intensive in terms of both computing power and cost.
- Solution: Start small with a pilot project. Many AI platforms offer scalable solutions where you pay only for what you use. Later, you can expand if you see a positive ROI.
Complexity in Explaining AI Decisions
- Challenge: Generative AI can be a “black box,” making it hard to explain why the model formed certain segments.
- Solution: Use explainable AI (XAI) tools that provide insights into which features influenced a segment or prediction. Pair generative AI outputs with simpler decision trees or rule-based models for validation.
5. Real-World Applications
E-Commerce
An online retailer might use generative AI to identify “seasonal shoppers” who make bigger purchases during holiday months. The AI can also analyze the language customers use in reviews—detecting those who mention “gift shopping” or “holiday deals”—and group them into a dedicated holiday marketing segment.
SaaS and Subscription Services
A subscription-based video streaming platform could create segments that anticipate which users might cancel based on a decline in watch time or negative sentiments expressed in feedback. The model can then generate personalized retention offers, like a temporary discount or recommendations for new shows, to keep these users engaged.
Travel and Hospitality
A hotel chain can collect data from loyalty program sign-ups, website searches, and customer service chats. The AI might identify a “business traveler” segment that values weekday stays, conference room bookings, and high-speed internet. It can then predict which new users are likely to fall into this segment and suggest a targeted marketing campaign with relevant perks.
Financial Services
Banks can use generative AI to segment users by their likelihood to apply for certain products—like mortgages or credit cards—based on transaction history, credit scores, and textual data from customer service inquiries. The AI can then generate content for each segment, detailing the benefits or application process in a user-friendly tone.
6. Best Practices for Success
Start with a Clear Use Case
Generative AI can do a lot, but it’s most effective when you give it specific goals. Focus on a single, well-defined use case—like reducing churn among mid-tier subscribers—and expand from there once you see results.
Combine Structured and Unstructured Data
By blending structured (e.g., numeric) data with unstructured (e.g., text) data, you can form a far more complete view of your users. This leads to richer segments and more accurate predictions.
Involve Cross-Functional Teams
Predictive segmentation isn’t just for the data team. Product managers, marketers, customer support, and even sales can offer unique insights into user behavior. A collaborative approach ensures the AI model is aligned with real-world considerations.
Maintain Ethical Standards
When predicting user behavior, always consider user privacy and potential biases. Offer easy opt-outs for users who don’t want to be part of advanced profiling, and be transparent about how data is used.
Continuously Update Your Model
User behavior evolves. Regularly retrain your generative AI model on the latest data to ensure your segments remain relevant. A segment that was effective six months ago might not hold true as trends change.
7. Measuring the Impact of Your Predictive Segmentation
Engagement and Conversion Metrics
- Open Rates / Click-Through Rates (CTR): For email campaigns targeting each segment, track if segmented messages perform better than generic broadcasts.
- Conversion Rates: Determine whether segment-specific offers lead to higher conversion compared to one-size-fits-all promotions.
Retention and Churn
- Churn Rate: After implementing AI-driven retention strategies for high-risk segments, see if your churn rate decreases.
- Repeat Purchases: Track how often users in certain segments come back to purchase again.
Revenue and ROI
- Average Order Value (AOV): See if predictive segments targeting high-value shoppers actually lead to larger transactions.
- Return on Ad Spend (ROAS): If you’re running ads specifically for AI-defined segments, measure the ROAS to gauge campaign effectiveness.
Qualitative Feedback
- Customer Surveys: Ask customers about their experience with your personalized offers or content. Do they feel the messaging resonates?
- Focus Groups / Interviews: Engage directly with users from different segments to get in-depth insights into the strengths and weaknesses of your segmentation strategy.
8. Future Trends in Generative AI for Segmentation
Real-Time Personalization
We’re moving toward an era where user segments are updated in real-time as behaviors change—imagine your AI model adjusting a user’s segment mid-session based on live interactions.
Multimodal Data Insights
Today, we focus on text and numbers. In the future, generative AI will draw from images, voice data, and even video, allowing for an even more holistic understanding of user preferences and behaviors.
Predictive Content Generation
AI won’t just identify the right segments; it will automatically generate entire marketing campaigns, from graphic design to copywriting, tailored to each segment. Some businesses are already experimenting with this.
Ethical and Regulatory Evolution
As AI’s capabilities grow, so too will the regulatory landscape. Expect stricter guidelines on how user data is used for predictive analytics, along with more robust user-consent mechanisms.
9. Conclusion: Making Generative AI Work for You
Implementing generative AI for predictive user segmentation can dramatically transform the way you market, sell, and engage with your audience. By combining the power of predictive modeling with the creativity of generative AI, businesses can gain a competitive edge—offering hyper-personalized experiences that delight users and drive revenue.
When approached with the right strategy and ethical considerations, generative AI becomes an invaluable partner in understanding and predicting user behavior. You’ll not only segment your users more accurately—you’ll also gain deeper insights into what drives them, allowing you to create personalized experiences that resonate and inspire loyalty.